The task of finding infringements across multiple channels requires a combination of tools, one of which should be image recognition technology. This is an important tool for finding fake items and copyrighted images online.
The rest of the brand protection checklist is covered in articles about machine learning, and how it can be applied to a brand protection service, and case management systems.
Intro to image recognition
Brand protection services are a must-have for any trademark holder or legal firm. Brand protection involves more than protecting sales and brand reputation; it is about safeguarding partners, investors and customers.
There are a number of brand protection providers in the market, and all claim to have comprehensive solutions. This article aims to provide decision-makers with a quick checklist of features and capabilities that any good brand protection service should contain.
Within this list, we will include aspects that consider usability, functionality and complexity of the systems. For this list, we will be comparing technology-based solutions only. Non-tech solutions, which don’t offer real online brand protection, are not sustainable and unable to react to modern day threat levels for brands. Given that few brands rely on traditional solutions, they will not be considered in this checklist.
What makes it smart?
Image recognition technology is not considered so cutting-edge these days. In fact, it is available to buy as a pre-made software. So, to evaluate a good image recognition feature, you must look at how that technology has been “trained” to identify images or products.
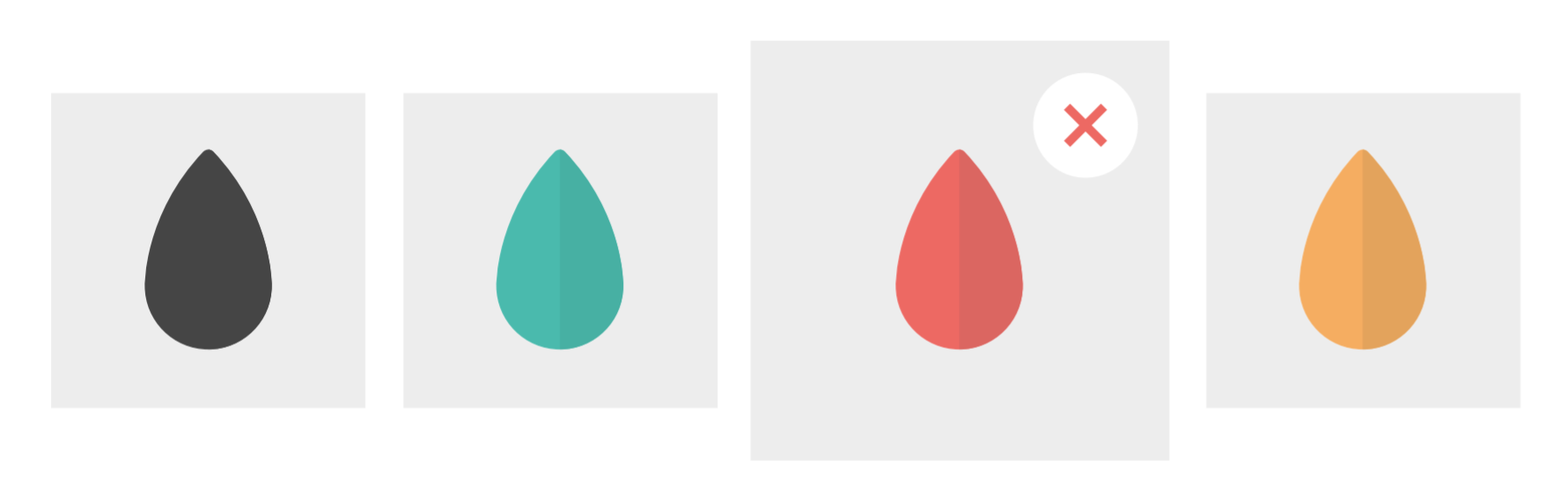
What is it used for?
Image recognition is used primarily to identify replicas. This is because design replicas do not tend to use the brand name of the genuine product, making them more difficult to find. Without image recognition tech, replica identification would have to be done by searching through product listings manually. However, depending on the client, image recognition can also be used to find potential counterfeits. Counterfeits tend to use the brand name in their description in some form. Therefore it is much more cost effective to search for counterfeits using keyword searches than image recognition, based on the processing cost.
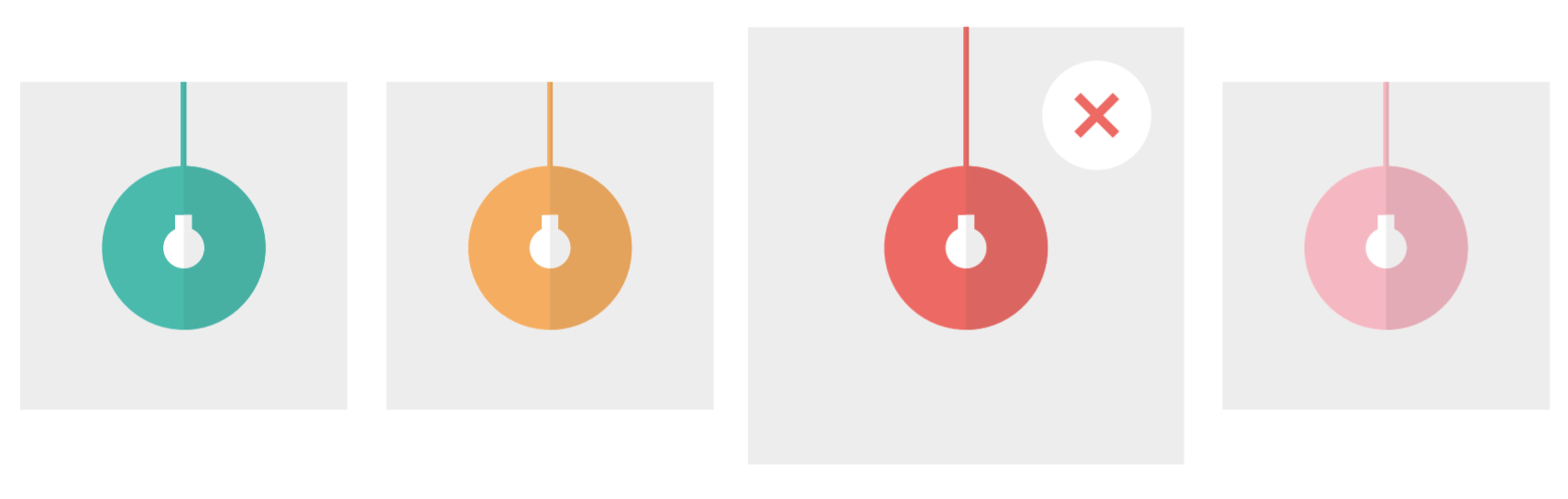
Is image recognition used for validation?
Image recognition tech is commonly used to find potentially infringing listings. However, the actual validation process is more complicated. Validation tends to be based on price, seller location, website and other factors. Machine learning programs may be able to create validation rules that are based on types of images or image features and other aspects of the listing, but image recognition generally isn’t used heavily to determine the authenticity of a listing.
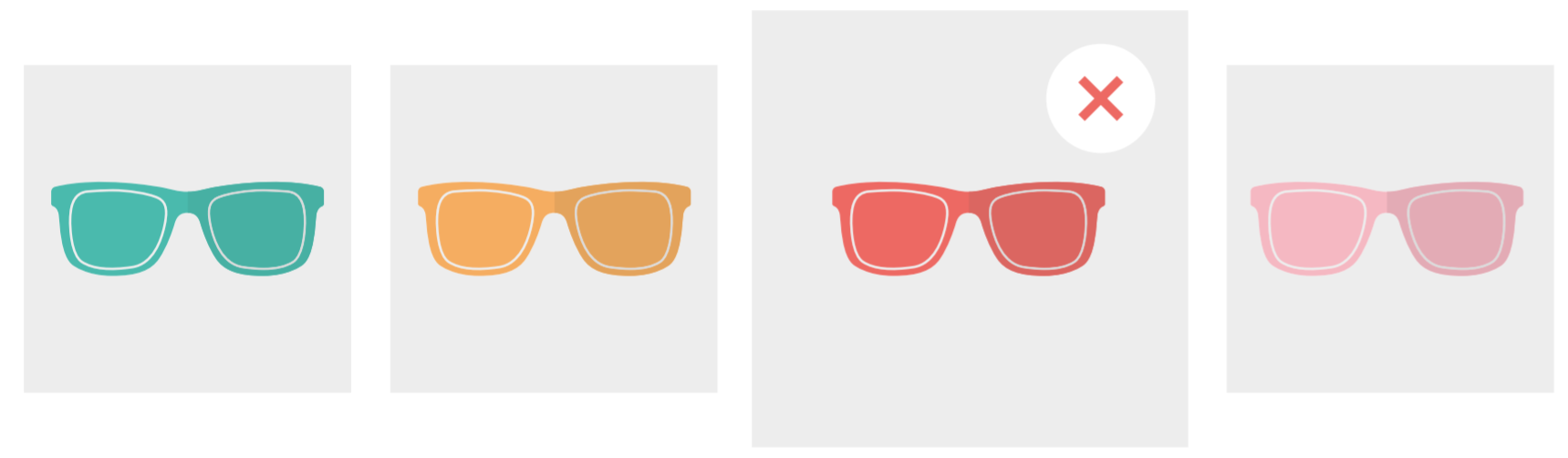
Photo recognition vs object recognition
Image recognition is a general term applied to all image-based identifying technologies; however, there are some important distinctions that must be made. Image recognition, in the case of brand protection, generally applies to technology that can recognise photos. This is helpful in identifying items that illegally use copyrighted images to sell unaffiliated products.
Photo recognition is relatively simple as the photo is unlikely to change much, although third parties can make minor alterations. However, as the photos tend to be the same, image-recognition technology doesn’t need to consider so many elements.
Object recognition, however, is more complicated. This is the process in which a program can identify a product within any photo or image. This involves being able to identify shapes, dimensions and other elements.
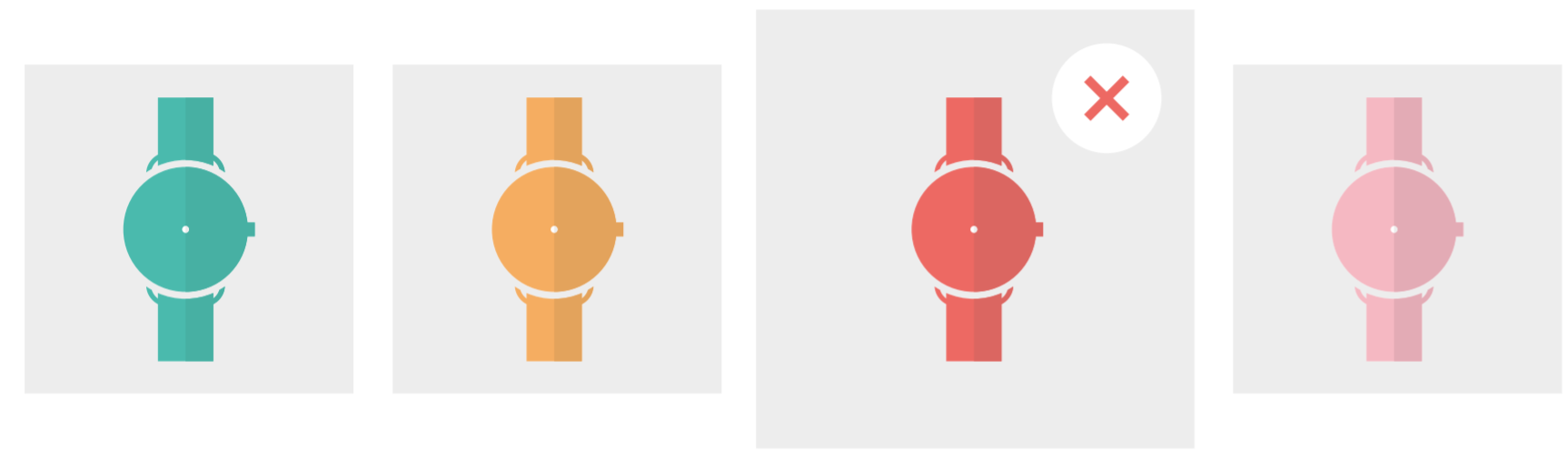
Logo recognition
Logo recognition is another aspect of image recognition technology. This, like object recognition, is the process in which a program can identify logos within images. By scanning an image, the program is able to identify logos, given their uniform pattern, and some suggest this is an easier task than object recognition. An excellent image recognition solution will use a combination of these tactics to create a full “image recognition” feature.
How do we combine these elements?
Scan every image?
The processing power that would be required to search through all the images on Amazon or eBay would be so high that any service doing so would have to charge substantial fees. Therefore, it is unlikely that any service does this, as it is neither an effective or efficient form of finding infringing items.
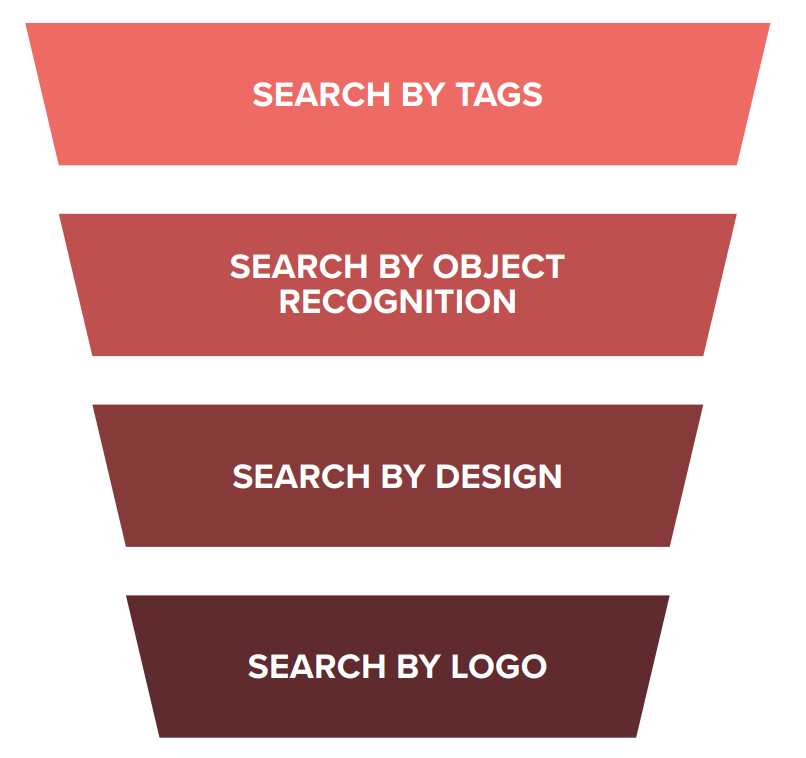
To illustrate this, we will use the example of finding counterfeit football shirts for “Manchester Example FC”. In this example, we will demonstrate one possible collection of image recognition features that could be used to protect the products of a client.
Funneling images
To find and accurately identify branded objects, an image recognition service will employ a funnelling technique. The exact procedure should differ depending on the product and brand, for reasons that will become clear.
Tags and keywords
The first layer of funnelling should be to select images that are tagged, described or categorised in a way that corresponds to the product. By selecting only listings from relevant product categories and with relevant keywords, the volume of images will be more manageable. This will immediately isolate all the associated pictures, saving time, processing power and, ultimately, money.
Example
To find our football items we would probably single out items that are tagged with keywords such as “Manchester Example FC”, “t-shirt” and other more generic descriptions like “Manchester sports top”.
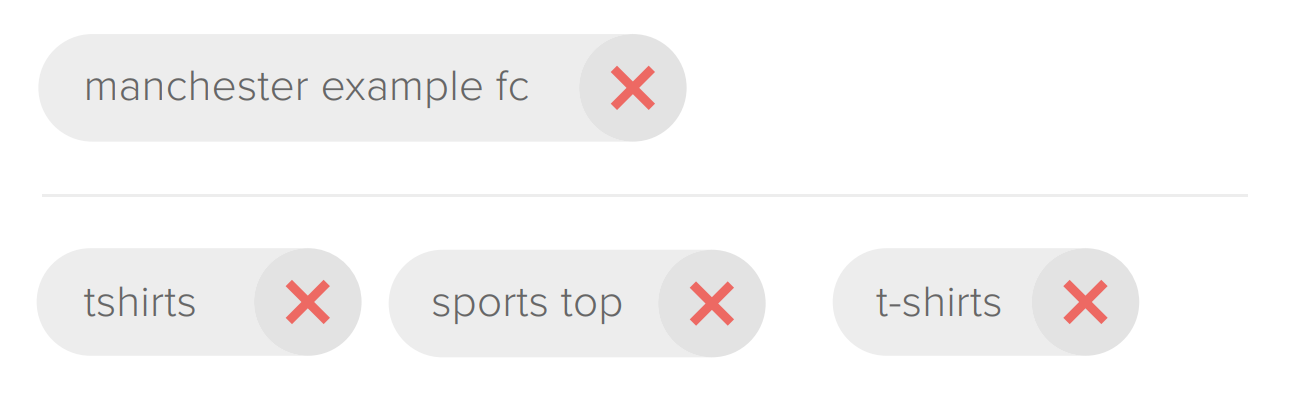
The second step is to filter out all the items that are not relevant to the search. A more sophisticated program would evaluate the images and decide if they match the essential elements required to be that product. As the process of removing counterfeits and having them return to ecommerce platforms goes on, counterfeiters learn to adapt their choice of keywords as they attempt to outrun brand protection services.
Example
To find t-shirts, the program must be able to analyse images and look for the essential elements that make up a t-shirt. These may be the dimensions of the product and key features such as a collar or sleeves.
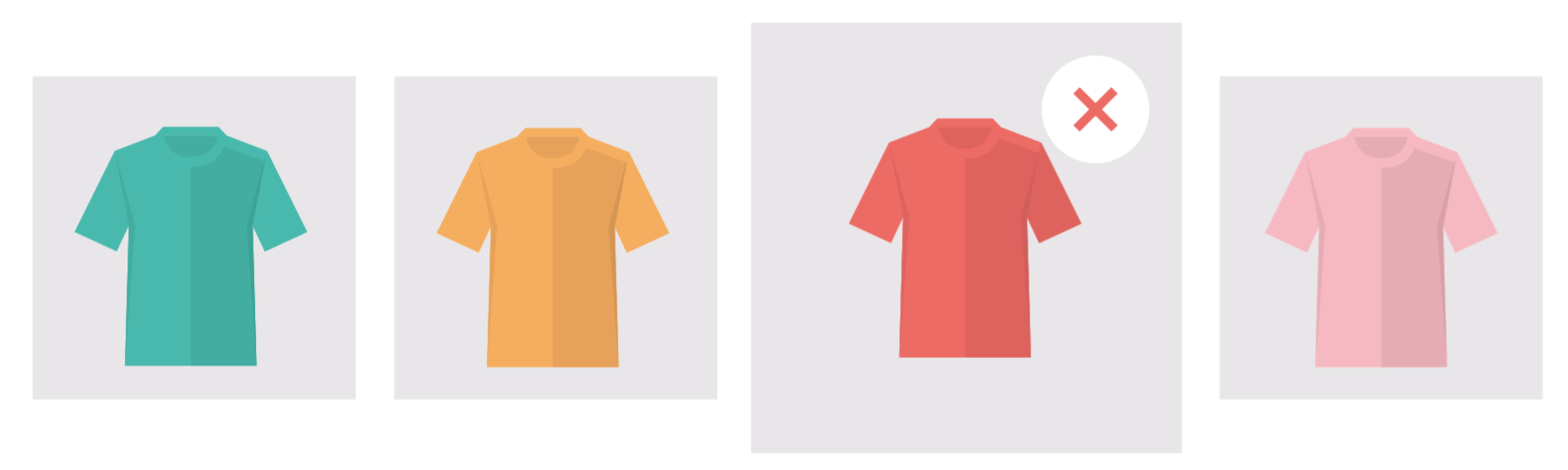
By design/product
The system is then presented with much fewer images. It must now work out if these objects meet the requirements to be considered the product in question. It can do this by analysing colours, layout and other design features.
Example
The system now has all the t-shirt items that are tagged using keywords relevant to “Manchester Example FC”; it must now analyse the t-shirts to see if they are indeed the football shirt we are looking to protect. Perhaps it will do this by searching for colour arrangement or stripes or anything that would make the item identifiable as the Manchester Example FC shirt.
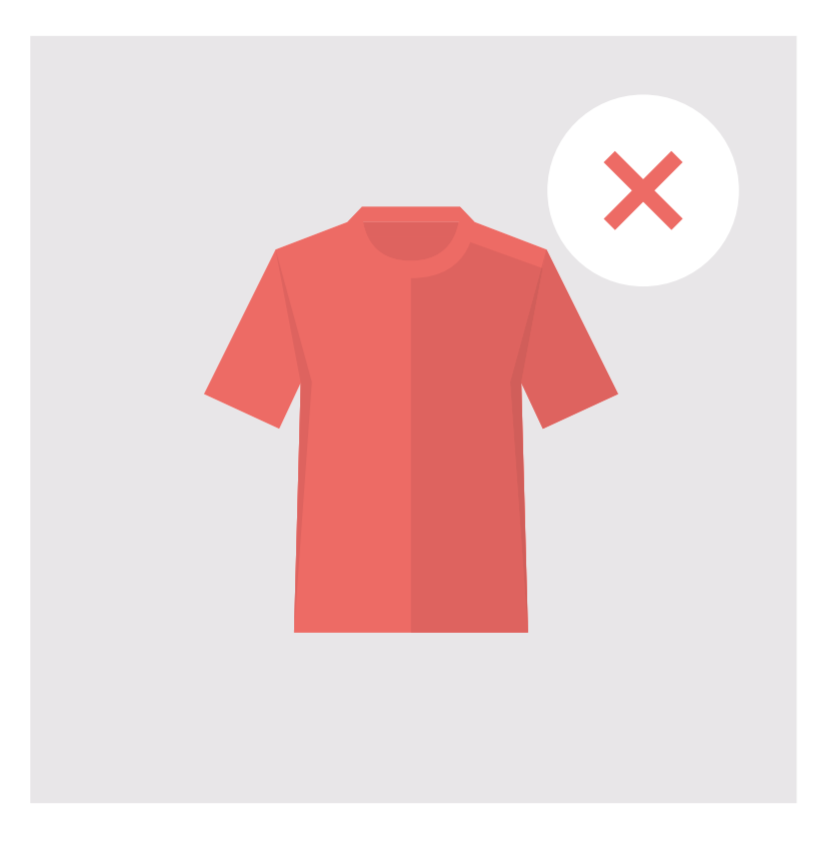
Logo detection
The system will now search within the confirmed product cases for a brand’s logo. This may differ as some items will be protected for their design and logos may not be relevant. Also, if a brand’s logo is unique, such as the emblem of a football club, then it will be relatively simple to detect on an image. However, if it is something more generic like Adidas’s three stripes, then it would be more difficult to find as these symbols are likely to appear in multiple locations. To make the process more efficient, the program would only search within set areas of an object or image, based on where the logo should feature. Again, this reduces the time and processing power required.
Example
The system now has all the items that match the design of the “Manchester Example FC” t-shirt. Logo recognition will search only 2 or 3 key areas of the image, such as the right/left the side of the chest or the top-left corner. These locations will be based on prior cases, where the logo is most often featured.
One size doesn’t fit all
Depending on the product, logo and type of infringement, the precise mix of elements will change. For example, the image recognition required for protecting a lamp design will be distinct from the image recognition strategy employed to protect a football club’s brand.
The more general the shape and form of the product, logo or design, the more difficult it is to safeguard via image recognition technology.
However, providing that the product has a characteristic that makes it discernable from other similar products, it can be detected using image recognition technology. But the more subtle the difference, the more significant the error margin.
The two most important things for image recognition are how it is onboarded or “trained” to identify objects, and secondly, how the technology can learn from previous cases to prevent substantial investments of time by staff.
Content in this article has been taken from the Image Recognition Ebook, available from the link below.
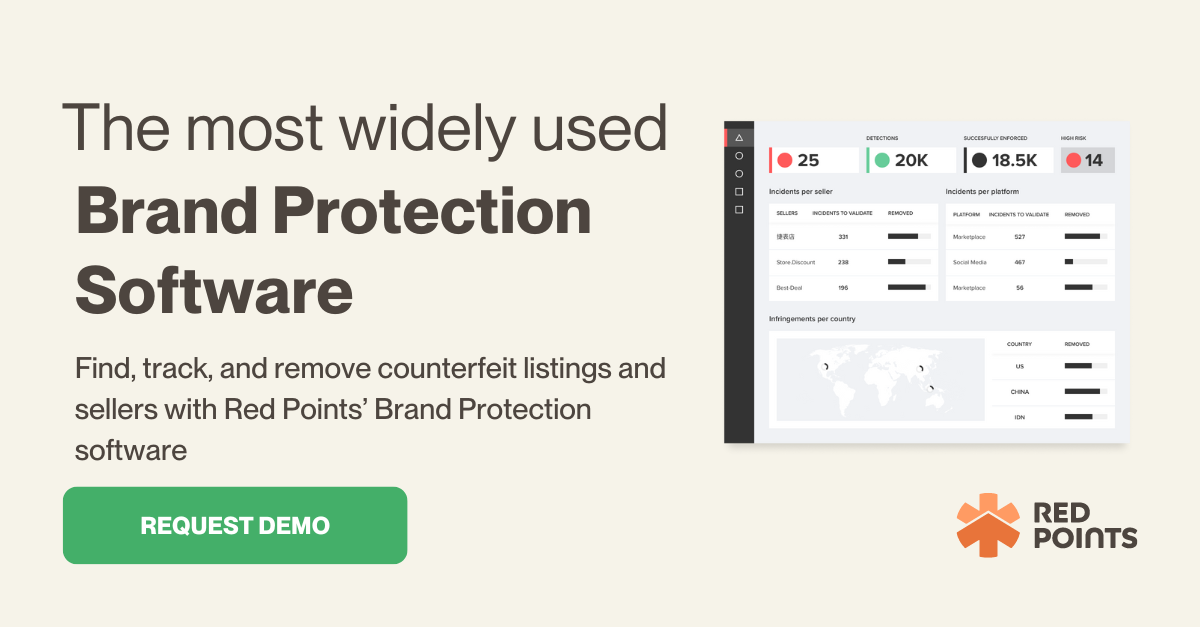